Transformer
Description
This API calls the Transformer model, which can be used for anomaly detection, classification, time series prediction, and forecasting.
Transformer Model Architecture
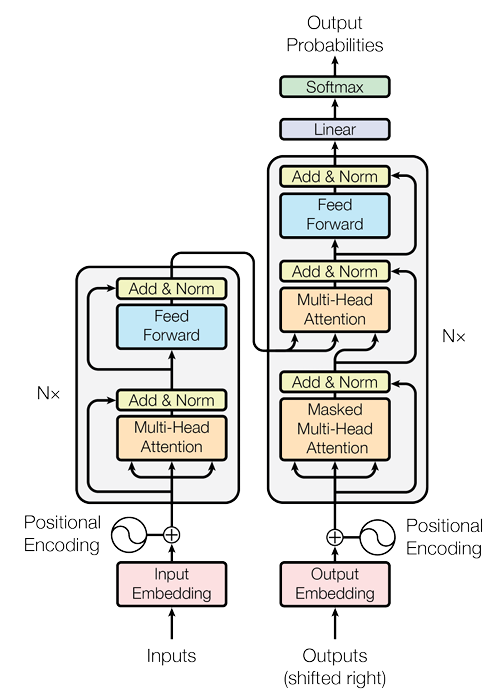
The Transformer is a powerful deep learning model initially introduced in the field of Natural Language Processing (NLP), but it is now widely used in various areas such as time series forecasting, speech recognition, and image processing. It was first presented in the 2017 paper "Attention is All You Need" by Google.
Key Features of Transformer:
-
Self-Attention
- Each word (or time step) in a sentence (or sequence) learns its relationship with other words (or time steps).
- For example, in time series data, the model learns how much a particular time step's value relates to values from the past.
-
Encoder-Decoder Architecture
- Encoder: Takes the input sequence and encodes it into a summary or abstract representation.
- Decoder: Generates the output sequence based on the encoded representation.
- In time series forecasting, both the input and output are sequences, with the decoder predicting future values based on the encoded information.
-
Positional Encoding
- Since Transformers do not inherently process sequential data like RNNs, they need a way to understand the order of elements in a sequence.
- Positional encoding is added to the input to provide information about the position of each element in the sequence.
-
Parallelization
- Unlike RNNs, Transformers process the entire sequence simultaneously, making them faster to train and more suited for parallel computation.
API module path
from api.v2.model.Transformer import Transformer, Transformer_Encoder
Parameters
input_dim
- Specifies the Input vector size.
- Example
- If data's shape (x,x,10).
- input_dim = 10.
model_dim
- Specifies the Model dimension.
- Example
- model_dim = 32.
num_heads
- Specifies the Number of attention heads.
- Example
- num_heads = 4.
num_layers
- Specifies the Number of Transformer layers.
- Example
- num_layers = 2.
output_dim
- Specifies the Output vector size.
- Example
- output_dim = 10.
dropout
- Specifies the dropout rate of layers.
- default : 0.2
- Example
- dropout = 0.2
Example Sample Code (Python)
Results
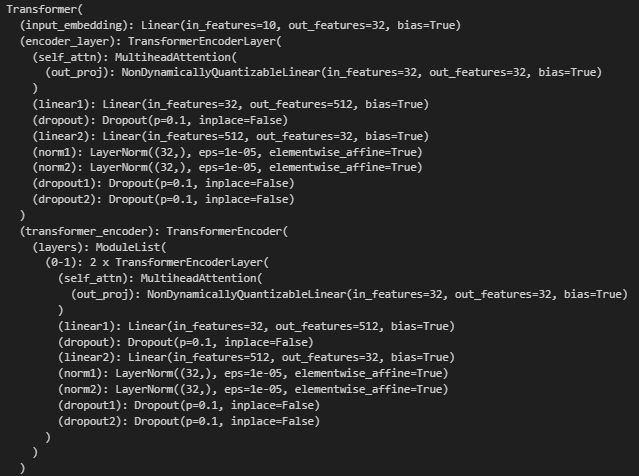
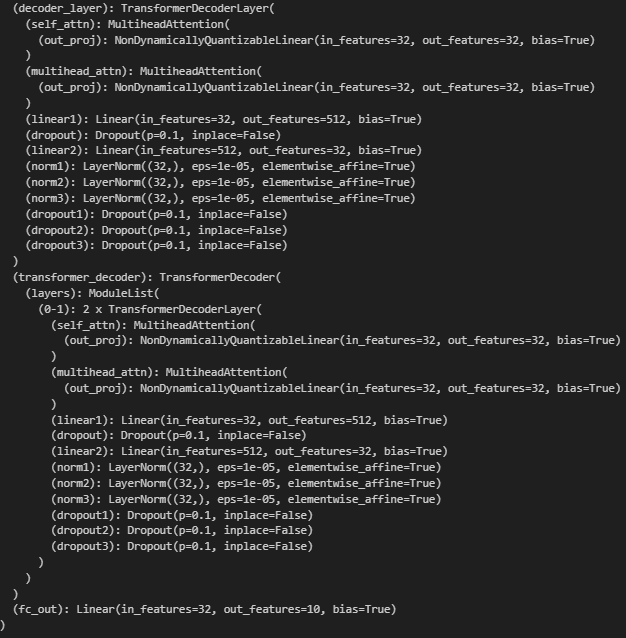
Check the entire module code.
datahub/api/v2/model/Transformer.py at main · machbase/datahub
All Industrial IoT DataHub with data visualization and AI source - machbase/datahub