Commercial Vehicles Sensor Data
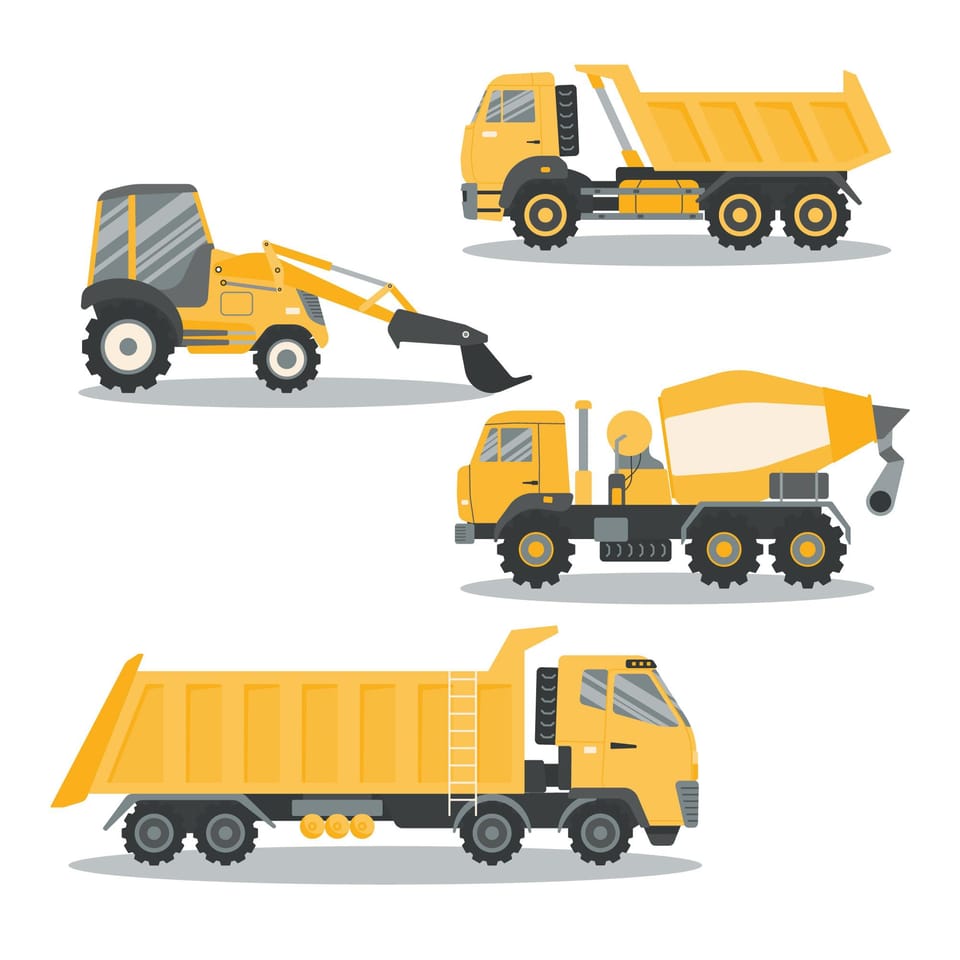
This post describes how to use Commercial Vehicles Sensor Data to Commercial Vehicles Status Classification through AI learning.
Table of Contents
- Data Introduction
- Data Visualization with Machbase Neo
- Table Creation and Data Upload in Machbase Neo
- Experimental Methodology
- Experiment Code
- Experimental Results
1. Data Introduction
- DataHub Serial Number: 2025-5.
- Data Name: Commercial Vehicles Sensor Data.
- Data Collection Methods: Collected through sensors installed on each dump truck, including accelerometers, speed sensors, and gyroscopes.
- Data Source: Link
- Raw data size and format: 156MB, CSV.
- Number of tags: 8.
Tag Name | Description |
---|---|
gFx | Force in X-axis direction |
gFy | Force in Y-axis direction |
gFz | Force in Z-axis direction |
speed | Speed |
wx | Angular velocity around X-axis |
wy | Angular velocity around Y-axis |
wz | Angular velocity around Z-axis |
label | 1 = Idling, 2 = Moving, 3 = Loading, 4 = Dumping |
- Data Time Range: 2025-01-01 00:00:00 to 2025-01-01 04:44:00.
- Number of data records collected: 13,599,864.
- CSV data URL: https://data.yotahub.com/2025-5/datahub-2025-5-commercial-vehicles.csv.gz
- Data Migration: Commercial Vehicles Sensor Data Migration
2. Data Visualization with Machbase Neo
- Data visualization is possible through the Tag Analyzer in Machbase Neo.
- Select desired tag names and visualize them in various types of graphs.
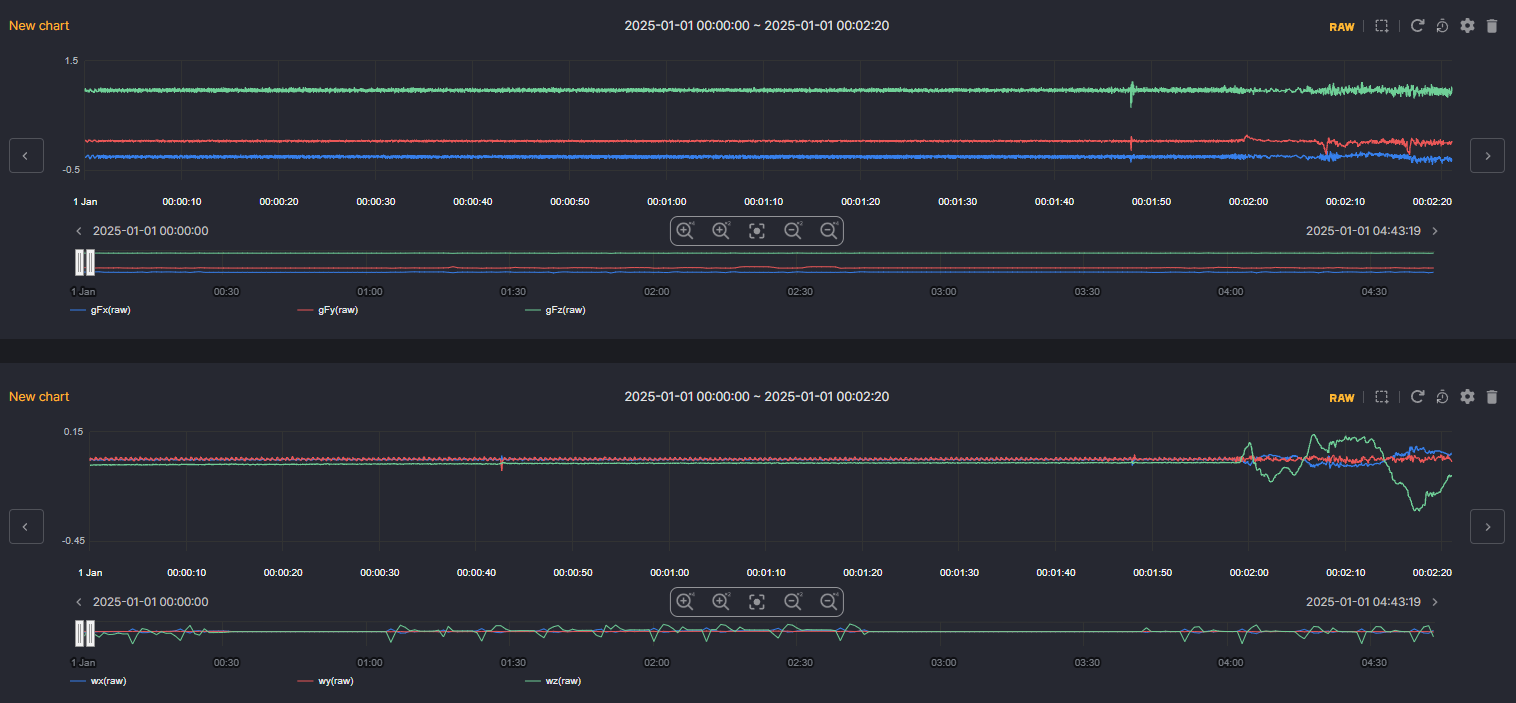
- Below, access the 2025-5 DataHub in real-time, select the desired tag names from the data of 8 tags, visualize them, and preview the data patterns.
DataHub Viewer
3. Table Creation and Data Upload in Machbase Neo
- In the DataHub directory, use setup.wrk located in the Commercial Vehicles Sensor Dataset folder to create tables and load data, as illustrated in the image below.
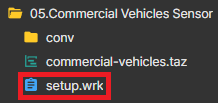
1) Table Creation
- The table is created immediately upon pressing the "Run" button in the menu.
- If the commercial_vehicles table exists, execute the first line and then the second. If it does not exist, start from the second line.
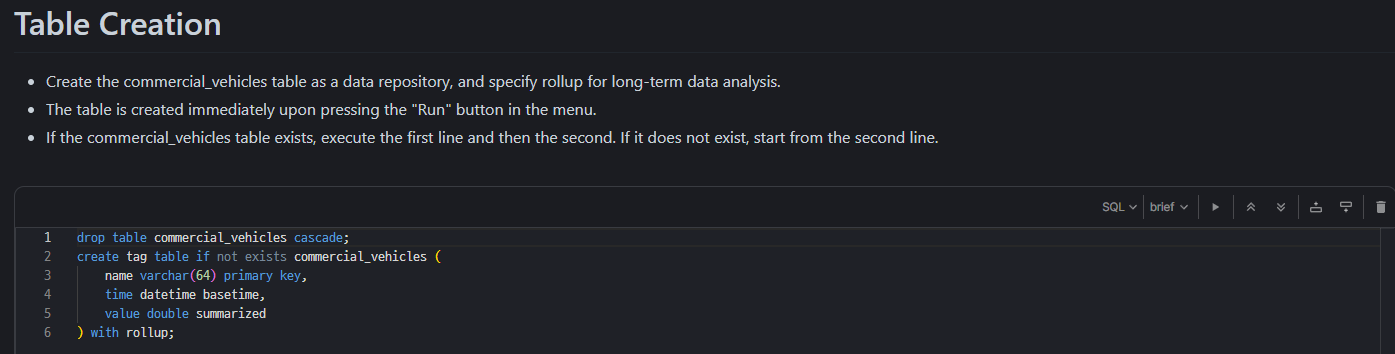
2) Data Upload
- Loading tables in two different ways.
Method 1) Table loading method using TQL in Machbase Neo (since machbase-neo v8.0.29-rc1
-
Pros
- Machbase Neo loads as soon as you hit the launch button.
-
Cons
- Slower table loading speed compared to other method.
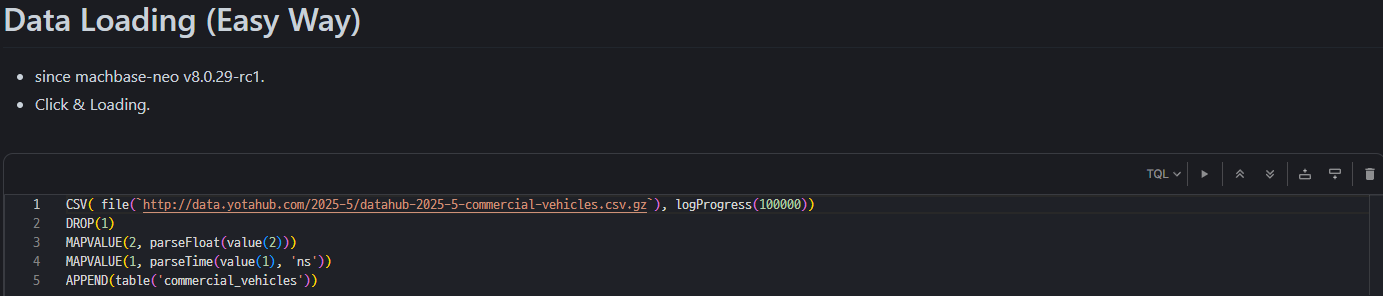
Method 2) Loading tables using commands
-
Pros
- Fast table loading speed.
-
Cons
- The table loading process is cumbersome.
- Run cmd window - Change machbase-neo path - Enter command in cmd window.
- If run the below script from the command shell, the data will be entered at high speed into the commercial_vehicles table.
curl http://data.yotahub.com/2025-5/datahub-2025-5-commercial-vehicles.csv.gz | machbase-neo shell import --input - --compress gzip --header --method append --timeformat ns commercial_vehicles
- If specify a separate username and password, use the --user and --password options (if not sys/manager) and add the options as shown below.
curl http://data.yotahub.com/2025-5/datahub-2025-5-commercial-vehicles.csv.gz | machbase-neo shell import --input - --compress gzip --header --method append --timeformat ns commercial_vehicles --user USERNAME --password PASSWORD
4. Experimental Methodology
- Model Objective: Commercial Vehicles Sensor Classification.
- Tags Used: gFx, gFy, gFz, label, speed, wx, wy, wz.
- Model Configuration: Transformer.
- Learning Method: supervised Learning.
- Train: Model Training.
- Test: Model Performance Evaluation Based on Commercial Vehicles Sensor Classification.
- Model Optimizer: Adam.
- Model Loss Function: CrossEntropyLoss.
- Model Performance Metric: F1 Score.
- Data Loading Method
- Loading the Entire Dataset.
- Loading the Fetch Dataset.
- Data Preprocessing
- MinMax Scaling.
5. Experiment Code
- Composed of three methods.
- Data Information: Outputs general information about the data.
- Visual Information: correlation heatmap, plot, Decomposition about the data.
- Statistical Test: ADF Test, KPSS Test, PP Test, ljung box Test, Arch Test, VIF Test about the data.
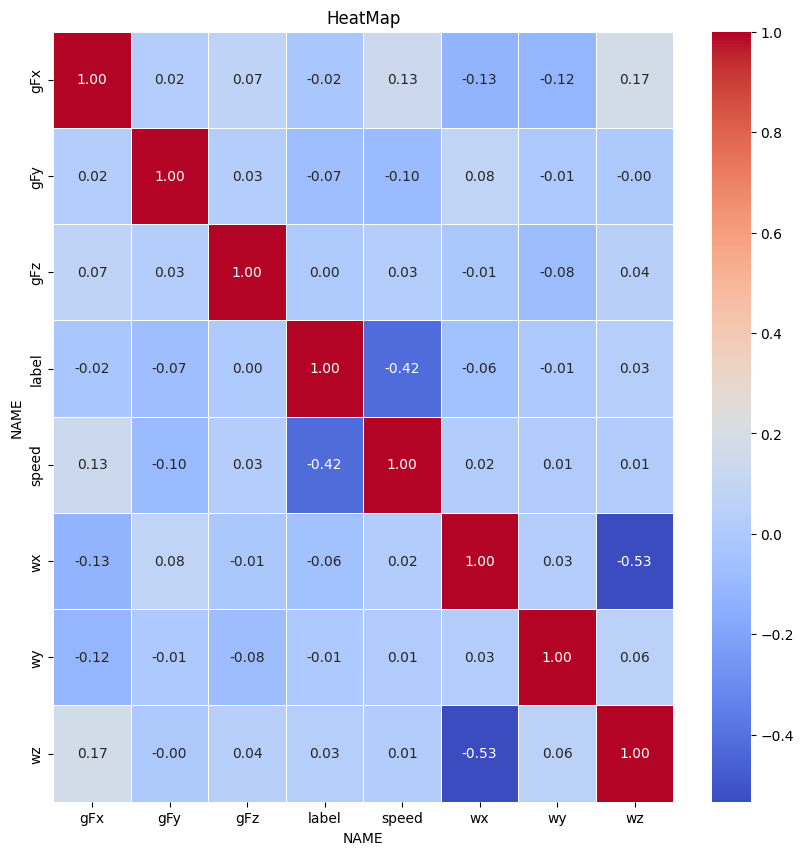
- The entire code can be run through 5.Commercial_Vehicles_Sensor_EDA.
Commercial Vehicles Sensor Classification
- Below is the code for each of the two ways to get data from the database.
- If all the data can be loaded and trained at once without causing memory errors, then method 1 is the fastest and simplest.
- If the data is too large, causing memory errors, then the batch loading method proposed in method 2 is the most efficient.
Method 1) Loading the Entire Dataset
- The code below is implemented in a way that loads all the data needed for training from the database all at once.
- It is exactly the same as loading all CSV files (The only difference is that the data is loaded from Machbase Neo).
- Pros
- Can use the same code that was previously utilizing CSVs (Only the loading process is different).
- Cons
- Unable to train if trainable data size exceeds memory size.
- The entire code can be run through 5.Commercial_Vehicles_Sensor_Full.
Method 2) Loading the Fetch Dataset
- Method for loading data from the Machbase Neo for a buffer size.
- Pros
- It is possible to train the model regardless of the data size, no matter how large it is.
- Cons
- It takes longer to train compared to method 1.
- The entire code can be run through 5.Commercial_Vehicles_Sensor_Buffered_Fetch.
6. Experimental Results
Method 1) Loading the Entire Dataset Result
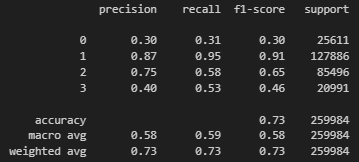
Method 2) Loading the Fetch Dataset Result
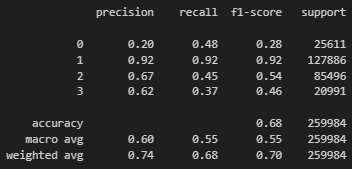
- The F1 scores of 0.58 and 0.55 indicate relatively low performance.
- There could be several reasons for the low performance, but they can largely be divided into two main factors.
- The first reason is that the data was collected without distinguishing between individual trucks, which undermines consistency.
- The second reason is that the data values do not differ significantly depending on the truck's operational state.
- Try improving the performance by applying various data preprocessing techniques!
※ Various datasets and tutorial codes can be found in the GitHub repository below.
datahub/dataset at main · machbase/datahub
All Industrial IoT DataHub with data visualization and AI source - machbase/datahub